CBIL: Collective Behavior Imitation Learning for Fish from Real Videos
Under Patent Review Process
Abstract
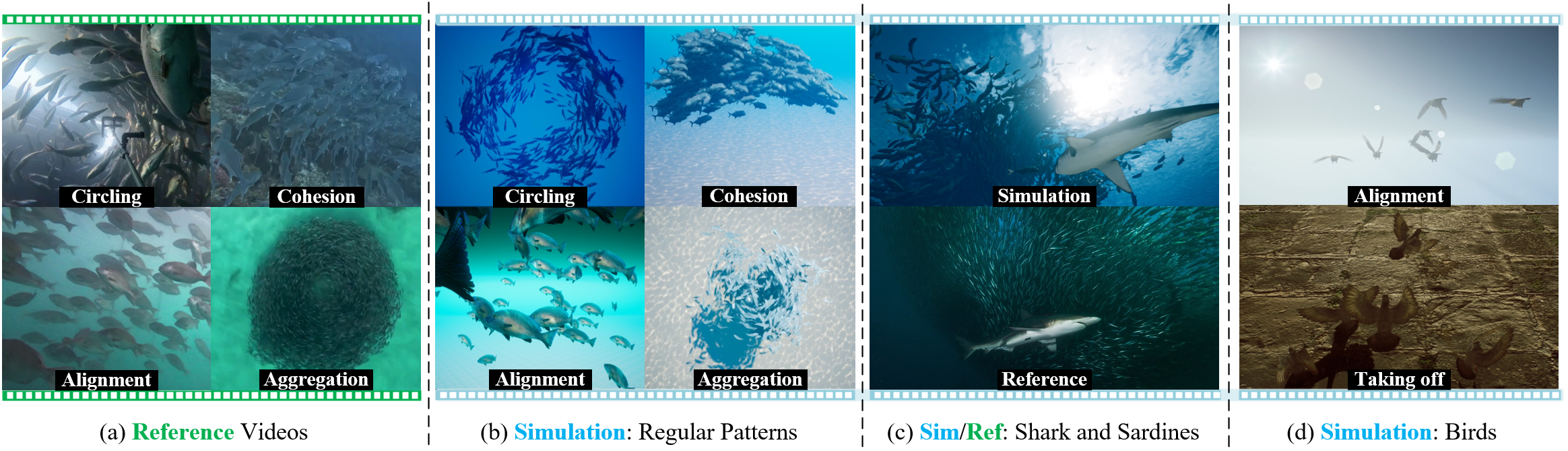
CBIL learns diverse collective behaviors of simulated fish from video inputs directly, enabling real-time synthesis of diverse collective motions. (a) reference video clips; (b) simulating varied behaviors of fish schools such as circling, alignment, cohesion, and aggregation; (c) fish schools responding to external changes and interactions; (d) motion control across different species, e.g., birds.
Reproducing realistic collective behaviors presents a captivating yet formidable challenge. Traditional rule-based methods rely on hand-crafted principles, limiting motion diversity and realism in generated collective behaviors. Recent imitation learning methods learn from data but often require ground truth motion trajectories and struggle with authenticity, especially in high-density groups with erratic movements. In this paper, we present a scalable approach, Collective Behavior Imitation Learning (CBIL), for learning fish schooling behavior directly from videos, without relying on captured motion trajectories. Our method first leverages Video Representation Learning, where a Masked Video AutoEncoder (MVAE) extracts implicit states from video inputs in a self-supervised manner. The MVAE effectively maps 2D observations to implicit states that are compact and expressive for following the imitation learning stage. Then, we propose a novel adversarial imitation learning method to effectively capture complex movements of the schools of fish, allowing for efficient imitation of the distribution for motion patterns measured in the latent space. It also incorporates bio-inspired rewards alongside priors to regularize and stabilize training. Once trained, CBIL can be used for various animation tasks with the learned collective motion priors. We further show its effectiveness across different species. Finally, we demonstrate the application of our system in detecting abnormal fish behavior from in-the-wild videos.
Framework
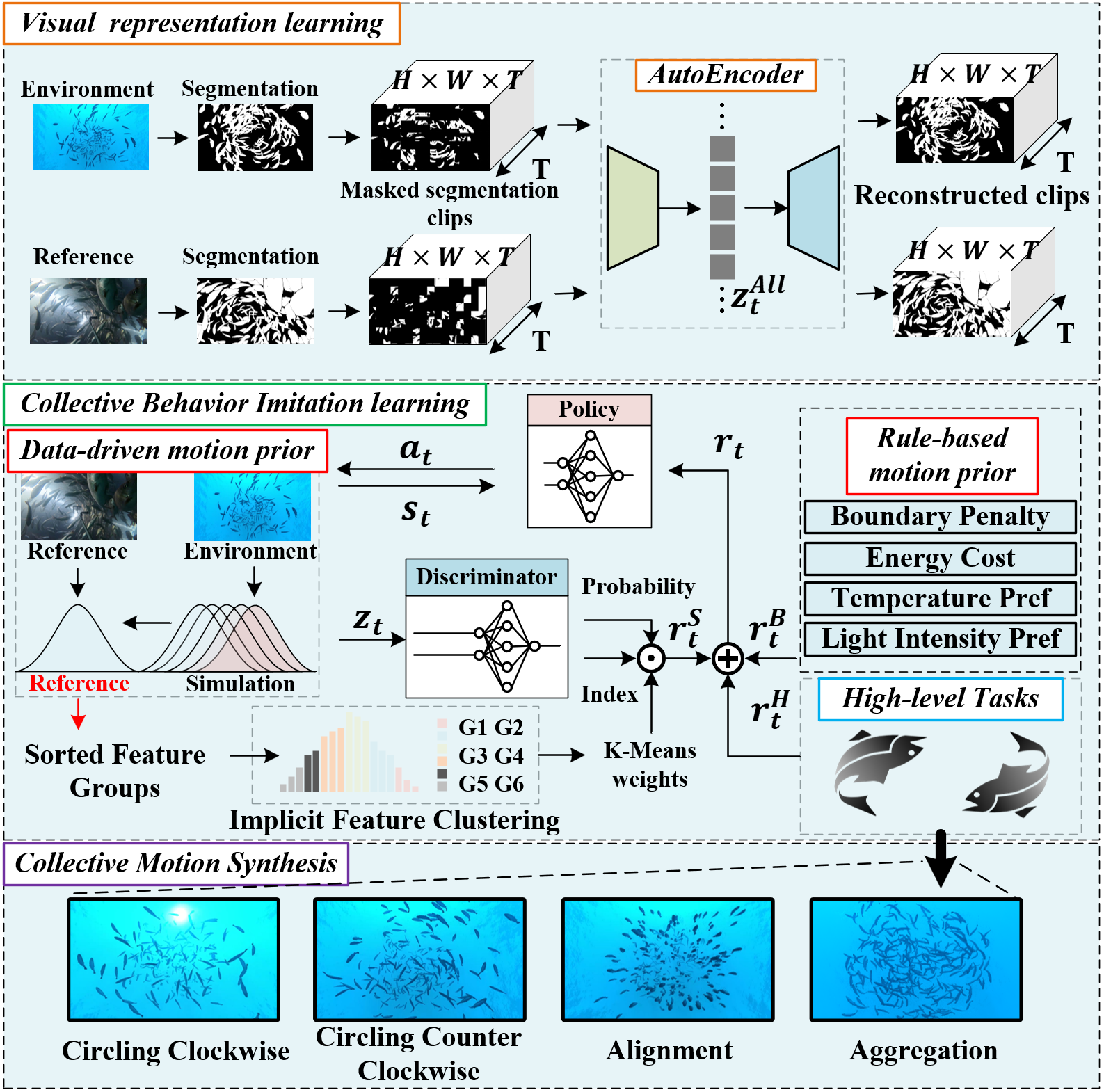
An overview of CBIL. Our framework has three stages: the visual representation learning stage, the collective behavior imitation learning stage, and the crowd animation stage for various animation tasks. In the first stage, we train the MVAE that learns mappings from video inputs to latent states. The latent states are later used in our collective behavior imitation learning. In the second stage, we employ both data-driven motion prior learned from videos and bio-inspired motion prior for imitation learning. Finally, we demonstrate that CBIL is applicable to diverse fish school animations such as circling, alignment, and aggregation.
Collective Motion Synthesis
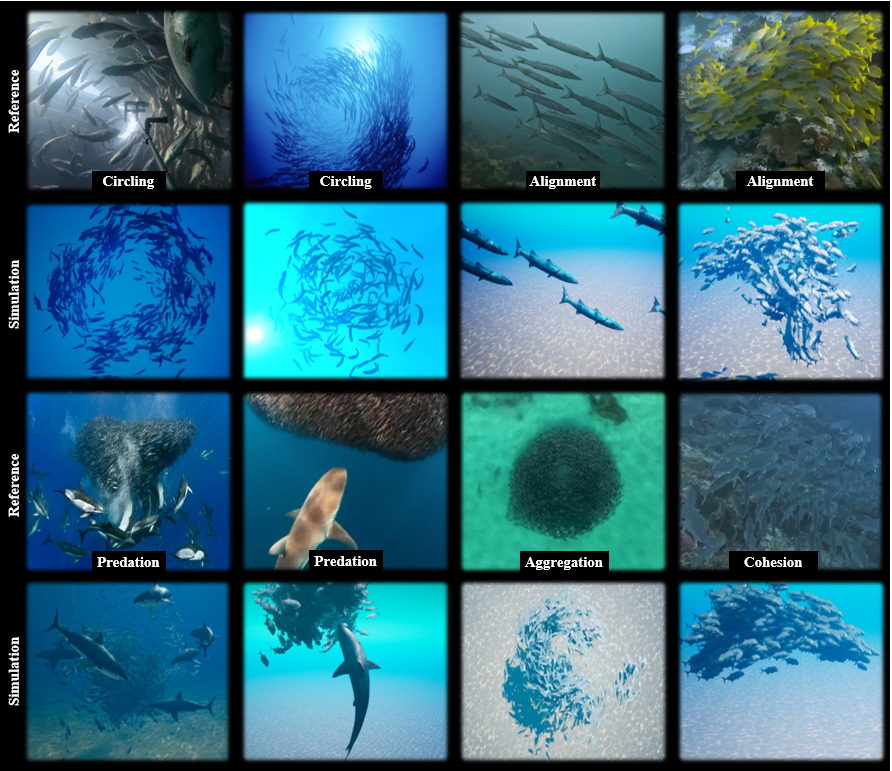
A gallery showcasing fish school animations reproduced using our method, highlighting its effectiveness across various fish species for reproducing diverse behaviors.
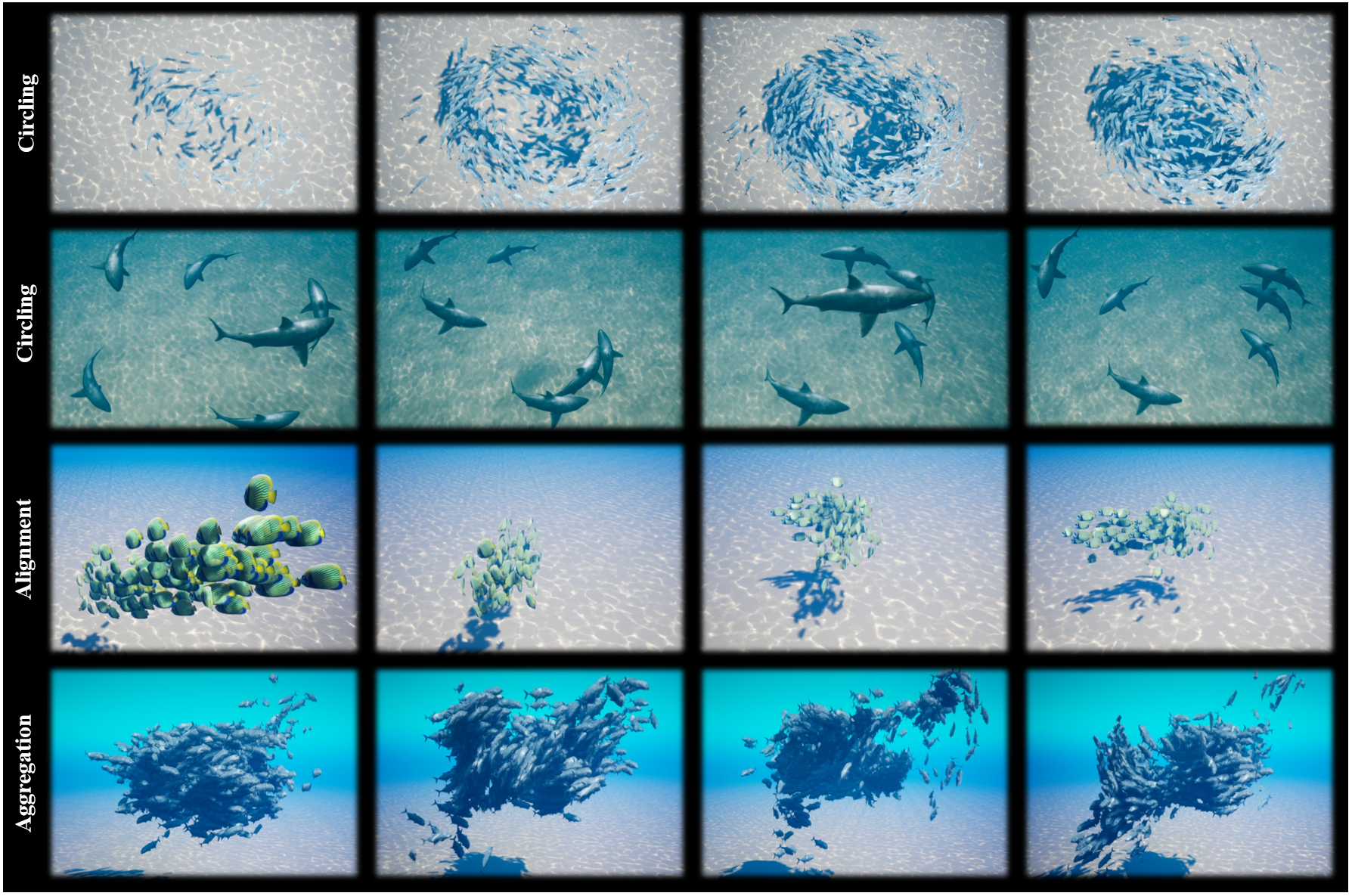
We showcase multiple animations of schools of fish with various movement patterns. (a) Tuna clockwise circling with 50, 100, and 300 fish counts; (b) Shark counterclockwise circling; (c) Emperor Angel Fish alignment with 50 fish counts; (d) Sardines aggregation pattern.
More Collective Behavior Patterns
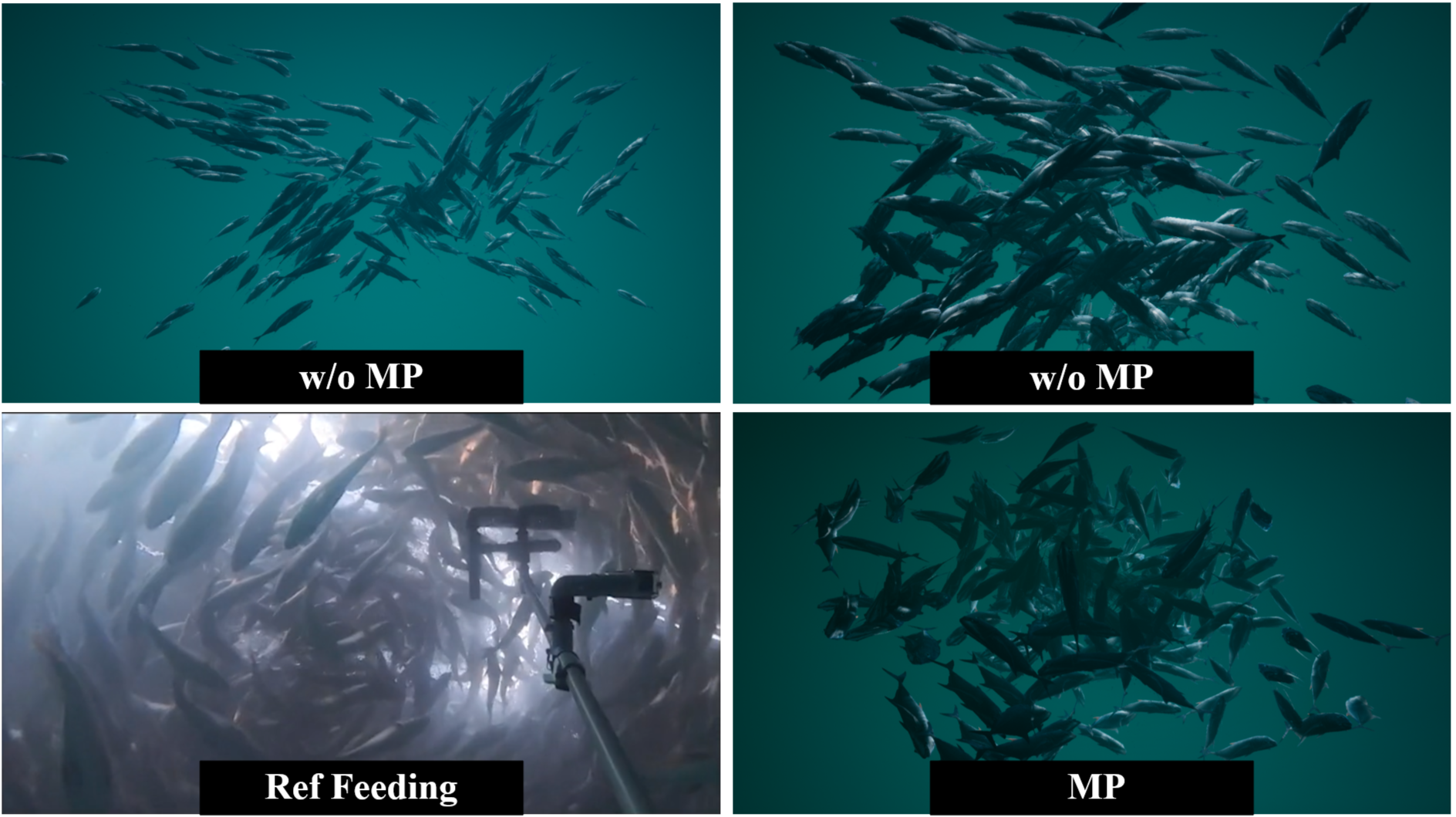
Validation of Motion Prior Effectiveness in Fish Feeding Task. Our method (bottom row), trained with video-based motion priors, produces a more realistic animation of the school of fish compared to the pure rule-based approach trained without motion priors (top row). In the simulation, food is randomly generated and disappears once the closest fish agent remains within its sensor range for 3 seconds.
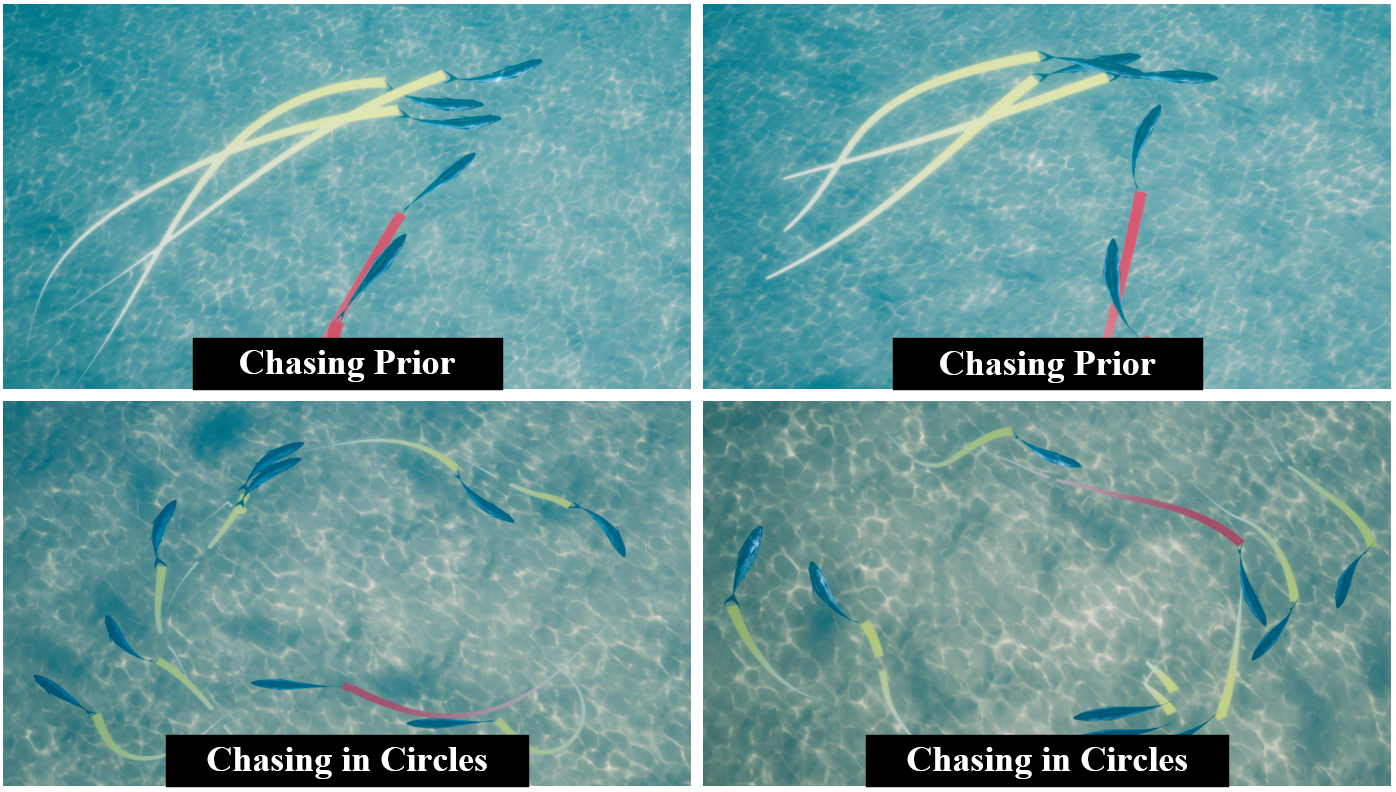
Chasing in Circles. The school of fish transitions from chasing behavior to a consistent circling pattern, with the dominant fish (in red) chasing the subordinate fish (in yellow).
Fish Abnomral Behavior Detection
Real-world data on fish behavior, particularly abnormal behavior indicative of environmental stress or disease, is not only scarce but also exceedingly difficult to capture due to the vastness and inaccessibility of aquatic environments. This motivates us to train a detection model using synthetic data that simulates abnormal behaviors, supplemented with a small amount of expert-annotated real-world data.
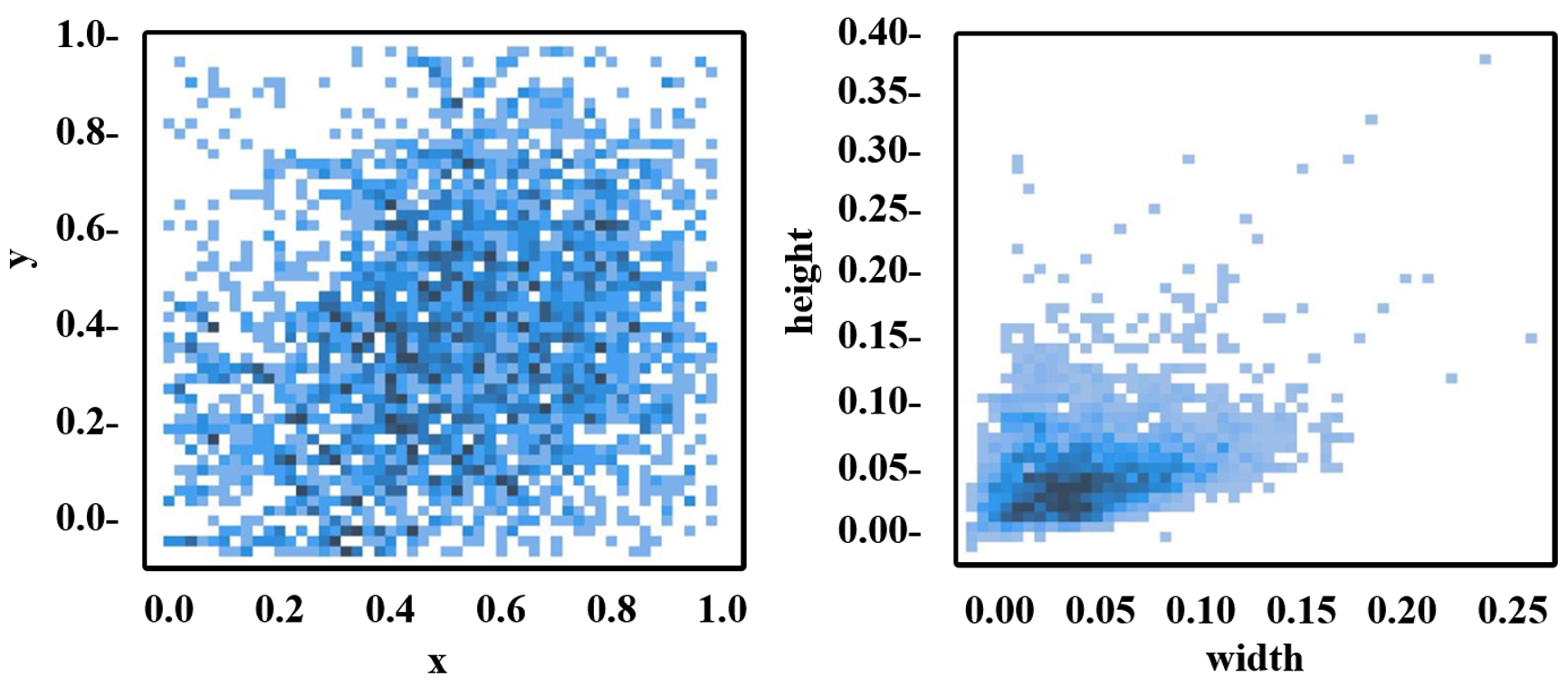
A total of 2901 fish are annotated in our training data, comprising synthetic and real images. We present the distribution of the bounding box locations and sizes, normalized by image size.
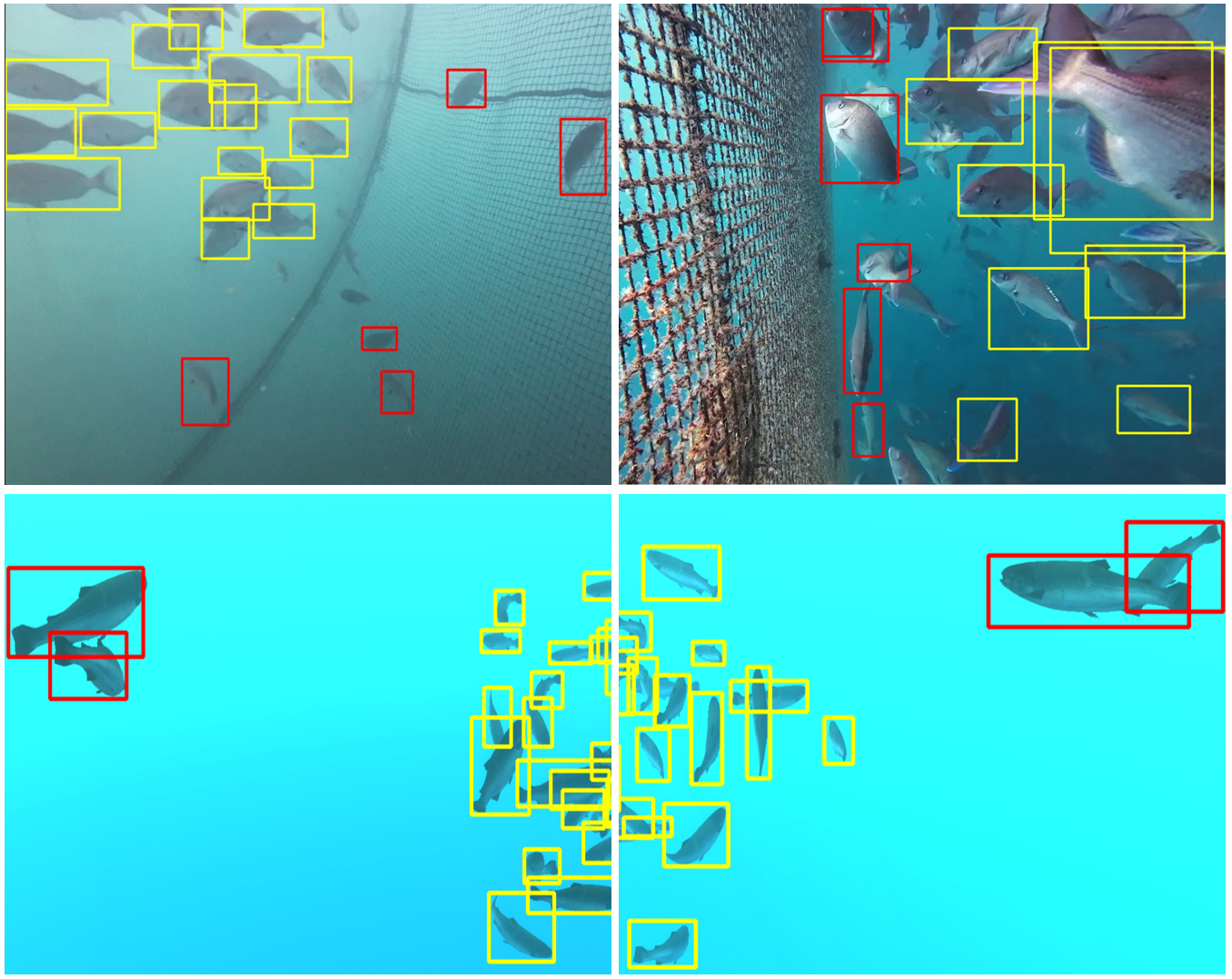
Samples of fish abnormal behavior detection from synthetic images and real images. The yellow box denotes normal fish while the red box denotes detected abnormal behaviors.
Generalization to Other Species
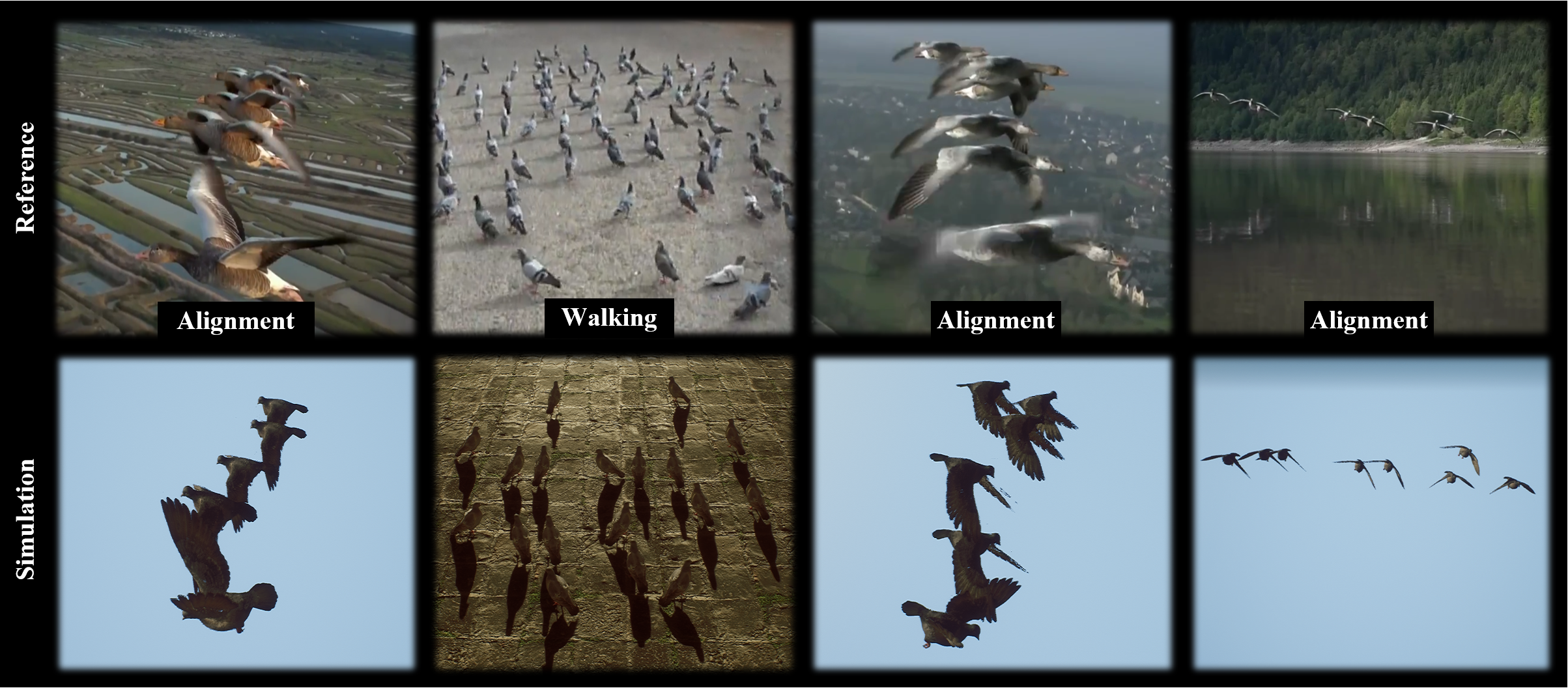
CBIL allows for crowd animation for different species. Here, we demonstrate the effectiveness of our method in simulating the flock of birds. Our framework reproduces a variety of behaviors learned from the reference videos.
Check out our paper for more details.
Citation
@article{wu2024cbil, title={CBIL: Collective Behavior Imitation Learning for Fish from Real Videos}, author={Wu*, Yifan and Dou*, Zhiyang and Ishiwaka, Yuko and Ogawa, Shun and Lou, Yuke and Wang, Wenping and Liu, Lingjie and Komura, Taku}, journal={SIGGRAPH ASIA 2024; ACM Transactions on Graphics (TOG)}, volume={43}, number={6}, pages={1--17}, year={2024}, publisher={ACM} }